Finance teams were the original data pioneers, with financial models serving as the earliest versions of metric trees. But, as data and metrics now permeate every aspect of the organization, our ability to create richer representations of business processes has expanded significantly.
Given their responsibility for monitoring the vital flow of funds within organizations, finance teams naturally led the charge in data investment and metric development. To this day, finance teams connect input metrics to core output metrics such as revenue, costs, and margins, tracking the monetary health of all organizations.
But, in the past decade, the data and metrics landscape has evolved dramatically. As various domains like sales, marketing, product, operations, and customer success have become increasingly specialized and analytically driven, there is an influx of operational metrics in these domains that ultimately influence and drive the financial outcomes.
However, due to the nature of the problem being solved, and tooling constraints (spreadsheets!), financial models have remained high level and relatively disconnected from the operational layer in many organizations. For instance, while a financial model may track metrics like new customer revenue by month or quarter, the operational side could involve numerous critical metrics and hundreds of segmentation cuts that drive this new customer revenue equation.
Modeling this increased richness of the business process via metric trees and bringing them to life can shape business strategy, and drive both financial and operational rigor.
The image below depicts a metric tree modeling gross profit over time from customer cohorts, illustrating how multiple metrics ladder up to total gross profit $ - from acquiring new customers (driven by the marketing function) to how these customers perform (driven by product, operations and customer success teams).
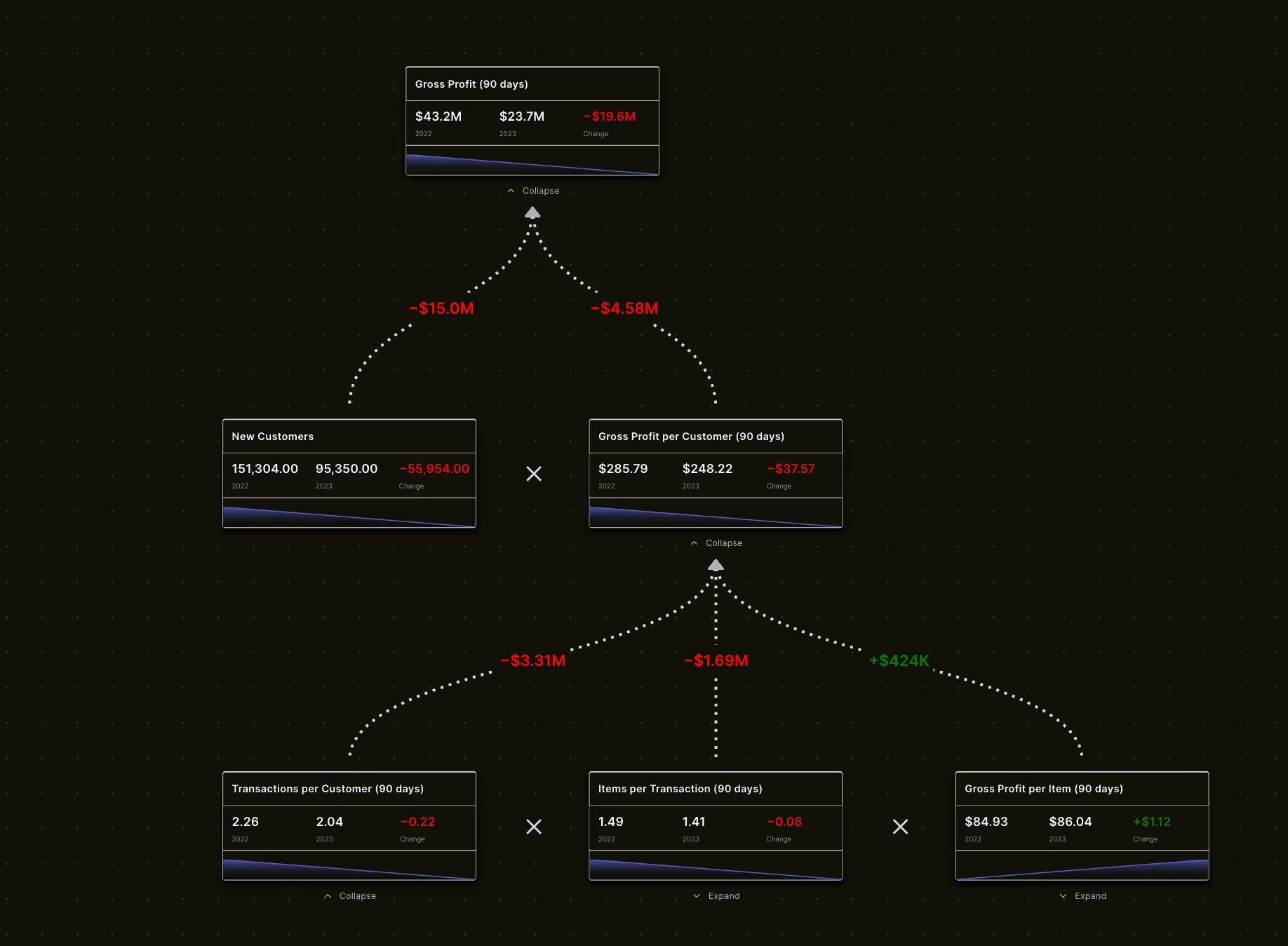
As you compare two time windows, you observe that the gross profit decrease is mainly driven by the struggles with absolute volumes of new customer acquisition versus the downstream performance issues per customer; and if you further decompose the downstream “per customer” behavior, you can observe that while retention metrics like transactions per customer are down, gross profit/transaction is actually improving thanks to the hard work of the operations and customer success teams.
One can imagine adding more layers of domain and segmentation depth to such a tree.
In essence, while financial models pioneered the concept of metric trees, modern iterations could capture far greater richness, and provide the ability to bridge the operational and financial worlds.